Conversational AI in finance changes how customers are served and how other businesses are conducted. This article discusses the advantages and disadvantages of conversational AI, as well as potential changes and forecasts, focusing on the banking and financial industry.
Current Statistics on AI in the Finance Industry and Future Prognosis
AI has especially gained broad acceptance in the finance industry due to the need to work more efficiently while serving customers. Between 2023 and 2024, the banking industry was the most active in AI investment. The stakeholders invested 20.6 billion US dollars.
Statista says that AI implementation is still at the preliminary level, though the financial services market is expected to have a big boost in the future.
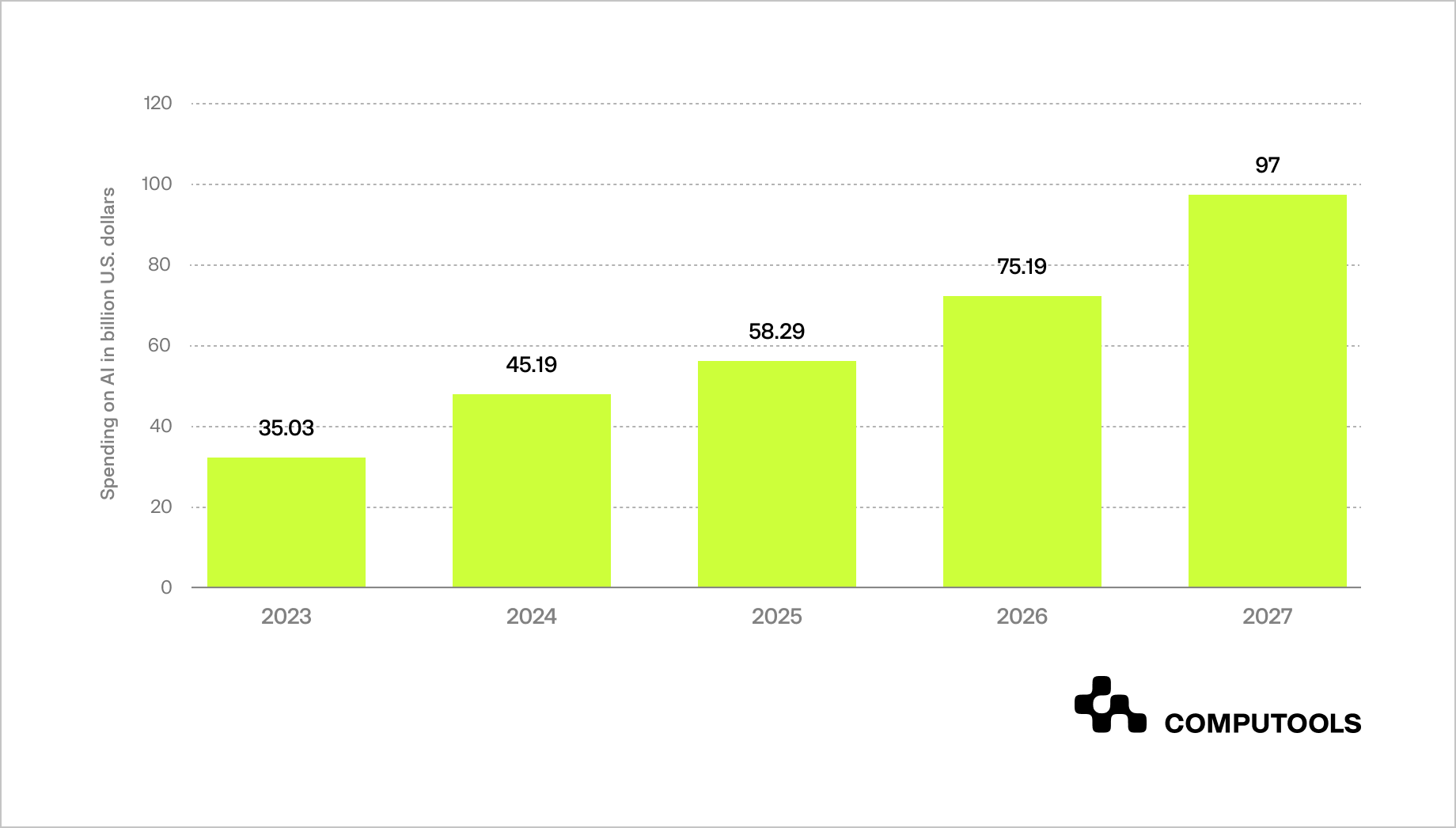
These findings capture the focus on the competition for AI investment and how, specifically, the financial sector can make the most of AI solutions.
AI expenditure in the financial industry is expected to rise significantly, with expected growth from 35 billion US dollars in 2023 to 97 billion US dollars in 2027. This means there was a compound annual growth rate of 29 per cent, predicting a steep rise in the investment in AI in the financial industry.
The use of AI in finance is developing swiftly. Two-thirds of finance executives are more confident in AI’s effect than a year ago, which is also positive, especially for those who have already made headway with AI solutions in finance.
See the current levels of AI use in finance:
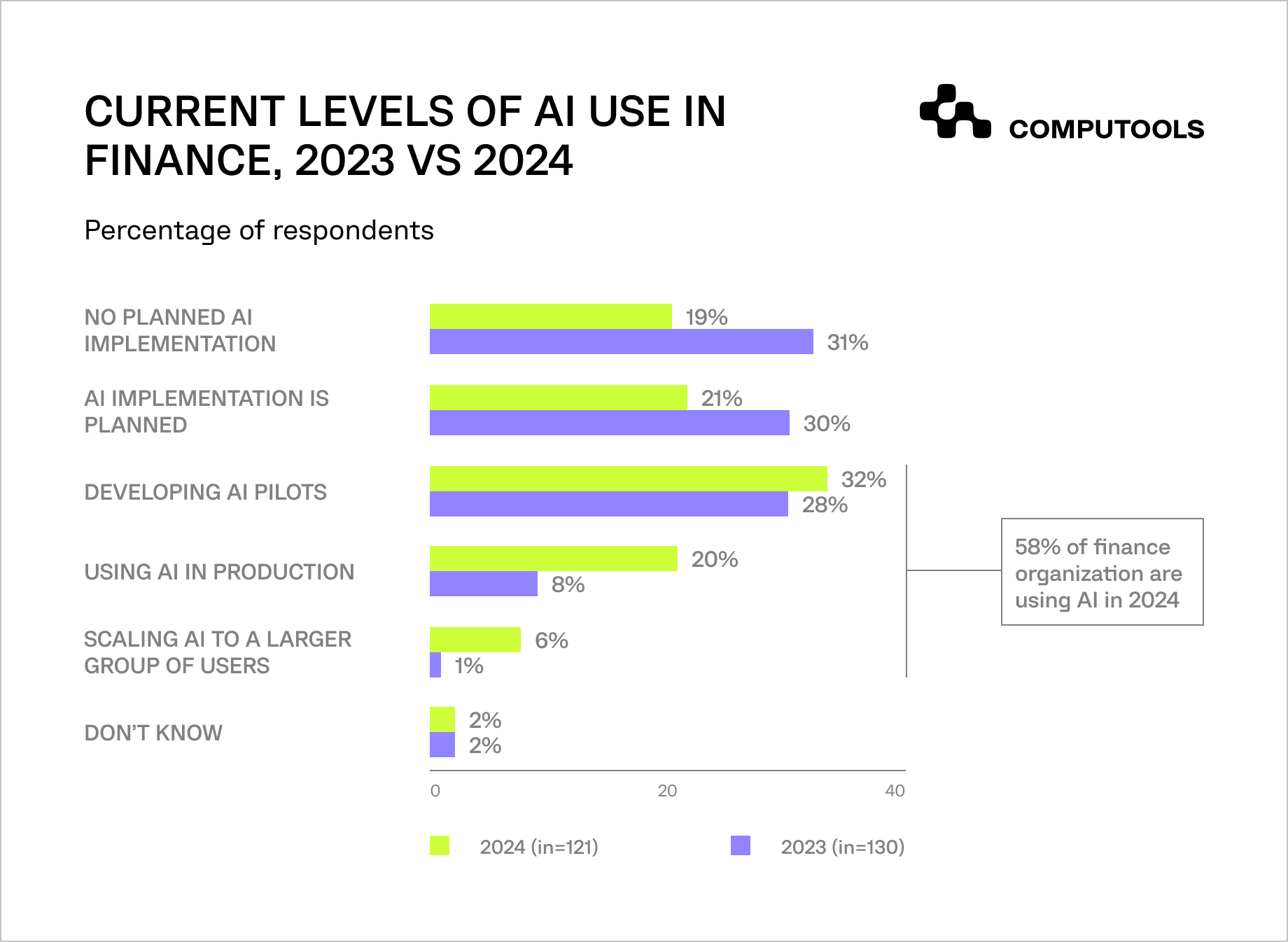
These trends highlight the rapidly increasing confidence and investment in AI within the financial sector, signalling a solid industry shift towards embracing advanced AI solutions.
As we delve deeper, let’s explore the essential role of conversational AI for the financial industry and why it has become a key focus for financial leaders worldwide.
Conversational AI: Definition and Its Role in Financial Industry
Conversational AI comprises solutions that facilitate interactions between man and machine using spoken or written language through natural language processing and machine learning.
The increased demand for clients in the financial industry due to emergent requirements for round-the-clock services, individualised advice, and fast operation with contracts rendered by bots can be regarded as the key value of conversational AI in banking.
The increasing demand for such capabilities has created the conversational AI market. Banking industry customers want convenient and quick methods of handling cash and other financial matters, debt and budget preparation, and fast response to their queries, which are making financial organisations adopt AI development services.
Core Conversational AI Technologies
Together, these fundamental technologies enable conversational AI systems that are flexible, dynamic, and able to replicate meaningful human interactions across a range of financial applications:
1. Natural Language Processing (NLP): NLP enables computers to translate human language by narrowing down text and spoken words into a machine-recognisable format.
Other subdomains of NLP are NLU, which enables conversational AI to understand the context, and NLG, which means the generation of valuable responses.
2. Machine Learning (ML): Conversational AI enables ML algorithms to allow the system to adapt and welcome improvements to previous and current performances.
This way, conversational AI, with the use of ML, will be able to improve its understanding of the preferences, language and contextual information that the user has provided during the conversation to make conversations intelligent and proper.
3. Speech Recognition: It transcribes speech, which allows a voice-mediated approach to a system. That’s why it works perfectly for virtual assistants like Alexa or Siri, which are based on speech recognition to capture users’ commands and provide relevant responses.
4. Sentiment Analysis: Using the receiver’s attitude, sentiment analysis assists conversational AI in steering its reactions to reflect or accommodate the user’s current feelings or temper to improve the customer experience.
5. Dialogue Management: This technology controls the way the AI talks and plans further reactions, such as input, previous conversations, and goals.
It makes the interactions relevant and structures the conversion to a particular direction, such as solving an issue raised or a transaction sought to be made.
Computools
Software Solutions
Computools is an IT Consulting and Custom Software Development Company that designs solutions to help companies meet the needs of tomorrow. Our clients represent a wide range of industries, including retail, finance, healthcare, consumer service, logistics and more.
Examples of Conversational AI
By streamlining procedures, improving client satisfaction, and facilitating real-time assistance, conversational AI in banking is revolutionising the whole sector.
It is utilised in the following important industries, particularly banking:
1. Customer Support
AI chatbots are taking over customer service systems by answering various consumer questions at any time. Among conversational AI use cases, there is Erica from Bank of America, who helps users monitor spending, pay for different payments, or check balances.
Erica may assist customers without speaking to a human agent who hogs the bank’s resources while meandering through decisions to make the customers happy.
2. Account Management
Through straightforward chat interfaces, conversational AI helps users carry out account-related actions, including moving money, checking balances, and reviewing transaction histories.
For example, customers may monitor accounts and get real-time warnings by sending text commands to Eno, Capital One’s virtual assistant. Thanks to this application, which simplifies account administration, customers may access banking more easily and quickly.
3. Fraud Detection
Real-time transaction pattern analysis using AI-driven systems can spot possible fraudulent activity. The technology may immediately notify the client through a chatbot or virtual assistant when it notices unusual activity.
By verifying suspicious behaviour from within their banking software development, HSBC helps consumers avoid illegal transactions by using AI solutions for banking to spot fraudulent tendencies.
4. Personal Financial Advice
Thanks to the abovementioned matters, chatbots and virtual assistants can analyse a customer’s spending and savings and propose individual recommendations.
For instance, due to analysing a customer’s spending, the AI chatbot developed by Wells Fargo can provide helpful tips on savings or spending. For this reason, users better perceive their finances and can make better financial decisions.
5. Streamlined Onboarding
AI solutions for banking make it easy for new users to set up their accounts and guide them through various legal requirements by offering to take photos of various documents.
Conversational AI is implemented in processes such as onboarding responsibility at JPMorgan Chase, where a lot of paperwork and documentation are reduced, and clients are helped to complete the process more efficiently.
This will not only help enhance the level of compliance but will also facilitate compliance-driven reductions in manual work effort when onboarding customers.
Benefits of Conversational AI in Finance
The implementation of AI solutions for banking offers numerous benefits, from enhancing customer experience to driving cost efficiency:
1. Enhanced Customer Experiences
Customers benefit from conversational AI because it provides instantaneous and customised answers to their questions.
For example, using AI-integrated chatbots lets Citibank be a more readily available resource to reach out to the customer base and resolve any potential issue, increasing customer satisfaction.
One advantage of successful products is that they better meet the current needs of customers; it enhances the image of a bank and increases its popularity among consumers.
2. Cost Efficiency
Reducing the amount of work at a given task and reducing operating costs associated with employing agents significantly decreases the costs associated with the job.
An example of its application is the large Spanish and multinational financial group BBVA, which launched an automated virtual assistant that handles frequent user appeals ahead of members of its team, thus freeing these officials up to deal with more complex problems.
This decreases overhead costs and increases the efficiency of resource usage, making the organisation leaner.
3. Scalability
Such an approach enables financial institutions to address hundreds of thousands of customer interactions daily, if not at the busiest periods.
For instance, when people have questions concerning taxes or times of stock volatility, companies such as the Goldman Sachs Group use artificial intelligence chatbots. This scalability will enable the bank to sustain high customer service metrics even during high-traffic periods.
4. New Methods of Data Collection and Data Analysis
Another potential of conversational AI is collecting customer data based on their interaction with the product or service. Lenders use artificial intelligence to study feedback on chatbots to improve their services and better address customer needs.
Using these AI development services, banks can then provide and enhance relevance to wanted and needed services.
5. Increased Security
Through machine learning, conversational AI provides flexibility in operations and continuous monitoring of unauthorised activities, thus enhancing security.
For example, conversational AIs at Santander help track deals and detect suspicious activity to prevent fraud. They can respond to customers’ suspicious activities immediately, adding security to the transaction.
Challenges and Solutions to Implementing Conversational AI
Despite its potential, several challenges hinder the successful implementation of conversational AI for financial industry:
1. Data Security: First, the security of customer data should be effectively ensured. In the modern world, many financial institutions deal with problems concerning privacy and GDPR compliance.
Solution: To enhance their security, AI systems should be equipped with strong encryption and access controls, which should be followed by regular audits.
2. Bias in AI Algorithms: This means that current AI systems can continue to enforce the bias in the data that feeds them, leading to unfair decision-making.
Solution: The third one is small recurrent checks on the AI results for biases and their correction, changing algorithms if needed.
3. Integration with Legacy Systems: Most organisations in the financial industry still use legacy systems that may not smoothly interface with new-age AI tools.
Solution: Devise on the development of API and the cloud to help in enhancing how old systems can integrate with conversational AI solutions.
Implementing Conversational AI in the Financial Industry: A Practical Guide for Businesses
Conversational AI is reshaping customer interactions and operational processes in the financial sector. As financial institutions continue to explore AI’s transformative capabilities, implementing conversational AI requires a strategic approach.
Below are key steps for businesses to successfully adopt and integrate conversational AI.
Key Steps for Implementing Conversational AI in Finance
1. Define Goals and Objectives: The first step is to define what conversational AI should do and achieve explicitly.
Although for some, using an interface for customer support or a convenient account management system may be a straightforward option, for others, it will be the detection of fraudulent activities. Anyway, being clear with your objectives from the very beginning will shape the development of the solution.
2. Select the Right Technologies and Models: When selecting AI technologies, it’s crucial to consider your company’s unique needs.
Whether it’s NLP, ML, sentiment analysis, or speech recognition, each technology should be chosen based on its ability to meet your financial service’s specific requirements.
Knowledge-based approaches can further enhance the AI system’s ability to understand context, refine responses, and adapt the communication process over time.
3. Integrate with Existing Systems: Many companies in the financial sector still operate in legacy environments.
Conversational AI must seamlessly integrate with these systems, enabling real-time data access and smooth transaction processing.
The majority of cloud services and APIs support the use of cloud infrastructure for AI systems, making integration relatively easy and ensuring the AI system is agile and capable.
4. Ensure Data Security and Compliance: To him, the most important thing is protecting customer data and following legal requirements in finance.
When using conversational AI, make sure your solution is encrypted, strictly controlled, and monitored constantly. Data privacy laws, including GDPR, should also be complied with.
5. Monitor and Adapt: Nevertheless, further performance analysis is required after implementation.
Monitor the AI’s behaviour with customers, fine-tune the equations used to utter responses, and modify the system to better align with the customers’ needs and market conditions.
Choosing an Expert Partner for Conversational AI Implementation
The effectiveness of conversational AI implementation is significantly impacted by how you choose AI software development company as a qualified partner with industry experience. Your AI solution will be tailored to satisfy industry-specific needs if you work with an AI development company that has demonstrated competence in the financial services industry.
Computools, for instance, specialises in AI software development for the financial industry, offering solutions that are perfectly suited to security standards, client happiness, and banking requirements. Our industry-specific expertise will give you the confidence that your needs will be met.
Our collection includes case studies demonstrating the effective use of conversational AI in financial contexts to help banks increase customer happiness, reduce costs, and streamline procedures.
For instance, one case study highlights how a Caribbean bank expanded into the younger demographic group and increased its market share by 12% in the 18-to-30-year-old segment by managing transaction validation and implementing parental controls and temporary blocks.
Our clients’ testimonials on how our solutions have improved their businesses are also available.
How Computools Supports Financial Organisations with AI Solutions
Our goal at Computools is to create conversational AI solutions in finance that tackle the particular difficulties faced by the sector. Our experience guarantees that every solution is safe, effective, and scalable, from fraud detection systems to AI-powered chatbots for customer service.
Look through our case studies in banking and finance to see how we have assisted customers in innovating and transforming their customer interactions with AI.
Financial institutions may collaborate with a reliable supplier like Computools to use our technological prowess and specialised experience to successfully negotiate the challenges of implementing AI and realise the full potential of conversational AI in finance.
Our proficiency in fintech software development may help your company maintain its lead in a cutthroat industry.
For a consultation on developing bespoke finance software and to begin improving your client experience, contact info@computools.com right now.
Computools was selected through an RFP process. They were shortlisted and selected from between 5 other suppliers. Computools has worked thoroughly and timely to solve all security issues and launch as agreed. Their expertise is impressive.