Artificial intelligence integration into the field of predictive analytics could be considered as a revolution in businesses, which is changing operations and optimising decision-making.
In this article, the current state of the AI market will be discussed; the concept of what predictive analytics means; how AI is connected with predictive analytics; the challenges that this connection may have; and examples of the use of AI with predictive analytics.
It will also offer information on future trends in predictive modelling and actionable business approaches for organisations in the adoption of AI in analytics planning.
Current State of the AI for Predictive Analytics on a Global Market
This is a crucial area because today more and more organizations make decisions based on the data they have. The possibility to analyze large amount of information, search for hidden connections and perform accurate predictions might be a powerful weapon on the hands of companies.
AI development services are rapidly accelerating, driven by substantial growth across various sectors.
Precedence Research estimates the current AI market will be approximately $638 billion in 2024 and is expected to reach $1.81 trillion in 2030.
On the basis of CAGR, the market is expected to grow with a CAGR of 38.1%.
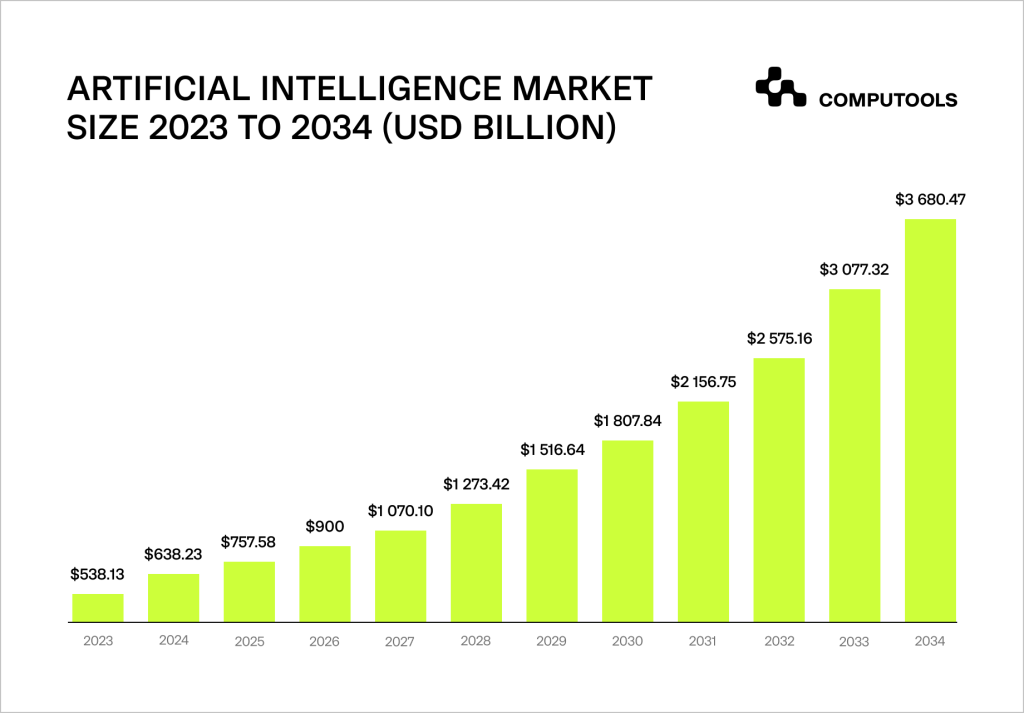
AI Adoption Growth Drivers
Market leaders including Google, Amazon and Microsoft, for instance, are increasing their spending in artificial intelligence indicating dynamism within the field.
AI is also increasingly becoming a business priority with 83% of firms believing that they need it to remain relevant.
The integration of AI technologies in various fields, particularly for predictive analytics, has been informed by several factors:
1. Financial Services
The use of AI and predictive analytics in the financial services industry mainly emphasises the capacity to strengthen decision-making procedures and optimise business performance.
The following factors contribute to its growth:
• Fraud Detection and Risk Assessment: Large datasets are processed by the use of AI in predictive analytics in financial institutions to enable the detection of fraudulent activities and undertake risk evaluations in a short span of time.
• Personalisation and Customer Experience: AI-powered predictive analytics can help banks deliver suitable financial solutions for customers by initiating spending behaviour and expectations, ultimately countering increased customer satisfaction.
• Operational Efficiency: Enabling computer-driven loan origination and client acquisition helps financial companies in three ways: it saves time, reduces mistakes, and decreases expenses.
These growth factors are a solid opportunity for banks because generative AI could contribute an additional $200 — $340 billion per year in operating profits.
2. Healthcare
In the healthcare sector, AI technologies are increasingly essential for predictive analytics, contributing to:
• Improved Diagnostics: Consequently, while AI applications may be able to analyze medical data they can diagnose pre-existing conditions than humans, therefore improving on the care that is offered to patients.
• Operational Efficiency: Implementation of technology in hospitals helps in performing a lot of clerical work that frees most of the personnel to attend to clients.
• Cost Reduction: In the application of AI, resource allocation is adjusted in such a way to reduce wastage and waiting time of patients among others.
AI application in healthcare is expected to trend upward continuously as it becomes a fundamental component of healthcare systems.
3. Retail
The retail industry has seen dramatic benefits from the adoption of AI, driven by:
• Enhanced Customer Insights: AI processes multiple-stream information to assist retailers in uncovering consumer trends to offer products accordingly.
• Operational Optimization: Automation of stock management and bespoke services like artificial intelligence suggested sales contribute to better performance and improving customers’ experience.
• Cost Efficiency: He or she can gather predictive insights for inventory which can help to reduce the expenses of holding stock and increase the company’s profit margin.
These innovations allow for heavy interaction with the consumer in terms of shopping as well as smoothing of their back-end processes.
4. Manufacturing
AI is crucial in the manufacturing sector, where its growth is driven by:
• Predictive Maintenance: AI predictive analysis enables manufacturers to predict equipment breakdowns even before they happen, therefore minimising on time wastage and the frequency of maintenance.
• Supply Chain Management: Through AI, firms achieve more supply chain transparency and flexibility so that they can respond to changes and needs expediently.
• Quality Control: Computerised applications maintain continuous supervision of the quality of products that are being produced to check if they conform to specified norms.
Accordingly, the reliance on artificial intelligence in production is expected to grow in a higher degree of market value because of technological advancements and the increasing implementation of integrated approaches within this application area.
Analytical data is a branch of business forecasting that uses information generated from past experiences together with statistical methods and business intelligence to estimate the likelihood of events happening in the future.
AI adds value to predictive analytics in that it offers superior analytical techniques and methods that increase prediction accuracy and reduce complexity.
As we see, people use AI not only in their workplace, but also at home:
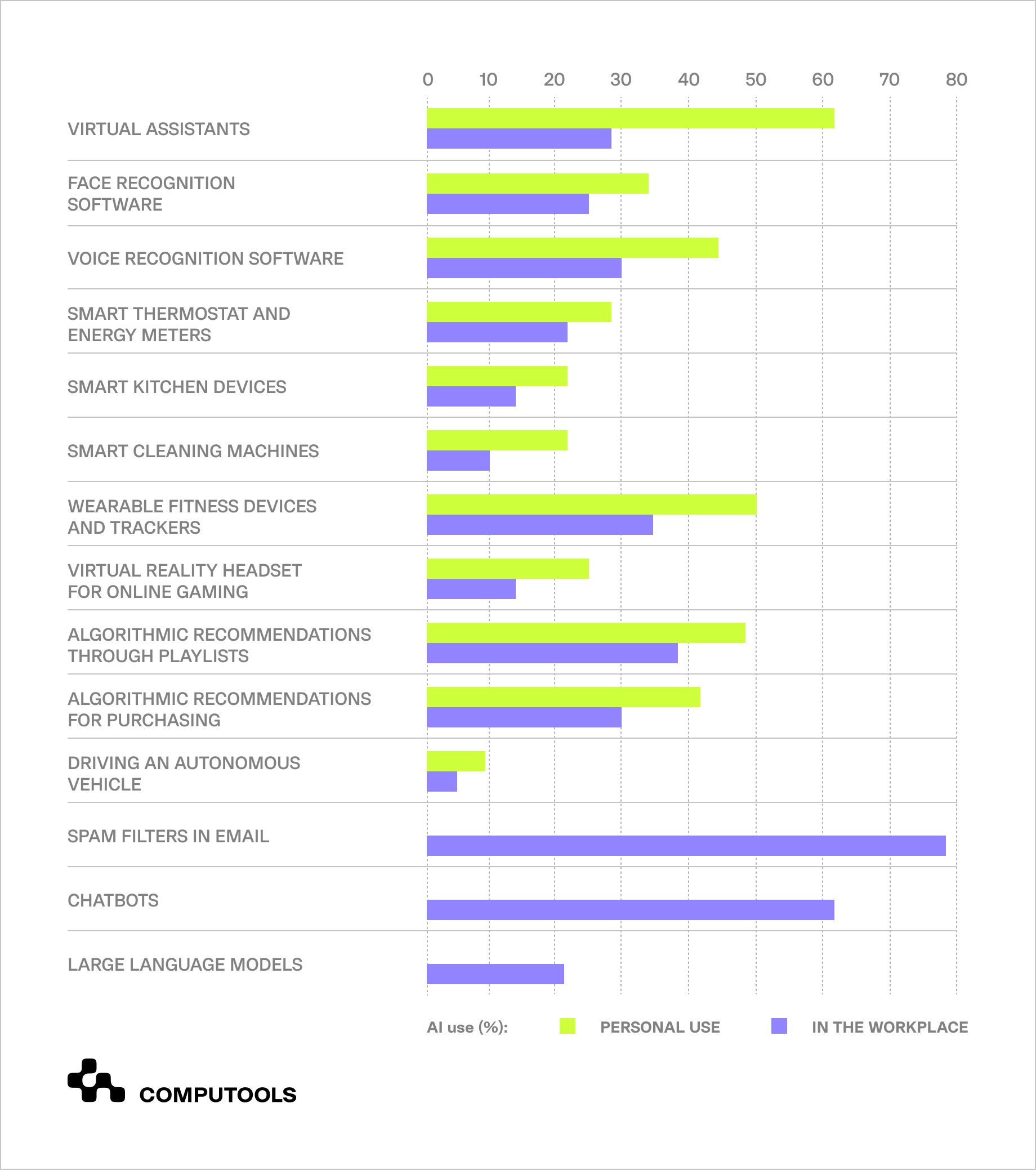
AI brightens the world of predictive analytics through using more sophisticated techniques like deep learning, ML and NLP.
Here’s how each contributes:
• Deep Learning: With the large amount of both structured and unstructured data, deep learning follows the intricacies, hidden patterns, and trends that conventional models rarely observe.
This enhancement is especially beneficial for those patterns which are subtle and complex like in customer sentiments or image diagnosis.
• Machine Learning: In AI predictive modeling, machine learning models, there is always input data with the ability of getting better and more accurate at the next prediction.
This make predictive analytics to transform depending on the recent data to cater for new trends in real time. ML facilitates a business to design a specific trend that is suitable for its operations like demand forecasting or risk evaluation.
• Natural Language Processing: NLP is a category in which text-based data is analysed and understood in order to create well formatted information out of the texts.
For customer service, sentiment analysis and feedback NLP is particularly valuable for companies to understand the message passed by a customer so that it can enhance its products and even advertise effectively.
AI predictive analytics for business leverages these technologies to improve decision-making and operational efficiency.
Key Benefits of Predictive Analytics for Businesses
AI has numerous advantages when incorporated into predictive analytics and these advantages result in improved value in a variety of organisational functions.
Predictive analytics empowered through the use of smart technologies helps refine prediction accuracy. The algorithms underlying its computation update what has been learned and make forecasts more accurate. This accuracy is valuable in sensitive areas like financial prediction and fraud control.
There is no need for human intervention as AI for predictive analytics automates data processing, leading to fast data analysis. This efficiency means faster rates of generating and processing information critical in enabling rapid decision-making suitable for dynamic business settings.
AI business software means that high-volume data, such as customer information, can be analysed at a superior rate and in real time. Future trends can be detected, allowing businesses to adapt to market shifts and customer behaviour. For example, real-time predictive analytics enable on-demand restocking of inventory since stock levels change in response to predictions of demand.
However, AI’s major strength is that predictive analytics can scale naturally with big data. This scalability is important for enterprises to gain insights about large data without exhausting resources.
Computools
Software Solutions
Computools is an IT Consulting and Custom Software Development Company that designs solutions to help companies meet the needs of tomorrow. Our clients represent a wide range of industries, including retail, finance, healthcare, consumer service, logistics and more.
Open Challenges and Limitations for AI Predictive Modeling
1. Current Limitations
Machine learning, specifically AI predictive analysis, is said to be a groundbreaking concept that is positively disruptive. However, its implementation is not without the magic levers that businesses must undertake in order to fully benefit from it.
AI-based predictive models, in many cases, need large volumes of data, a significant portion of which is always going to be PII-sensitive, as are most industries these days, especially those in the healthcare and financial markets. Data privacy and adherence to policies like GDPR or HIPAA continue to raise difficult issues.
Machine learning models are only as accurate as the information that has been fed to them. Demographic, geographical, and other forms of data bias result in poor prediction and decision-making, they argue. This bias is more alarming, especially in sensitive areas such as healthcare, where a wrong prediction can have life-changing effects.
Creating and deploying overarching PMs based on predictive analytics and AI prediction algorithms is technically demanding. As part of data preparation, model selection, and monitoring, the process should involve professionals from data analytics.
This has implications for increasing implementation costs, which are hard for some businesses to undertake and sustain the systems. Leveraging AI solutions for business can help mitigate these challenges by providing tailored insights.
Ultimately, investing in comprehensive AI business solutions can enable organisations to harness the power of predictive analytics while addressing the associated complexities.
2. Industry-Specific Challenges
Certain sectors face unique challenges in implementing AI-driven predictive analytics, where accuracy and precision are critical:
• Healthcare: This means that predictive analytics in healthcare must include high-risk factors like the diagnosis of patients and the treatments proposed. Health data is very sensitive and needs to be well-protected hence the implication of mistimed and wrong predictions which can be very costly to the patient.
Further, inaccuracy in training data creates biased conclusions for one kind of patient type as opposed to another kind.
• Finance: Business organisations use it for credit risk assessment, credit risk analysis, credit risk monitoring, and credit rating.
However, the variation in economic conditions, extreme volatility, and changes in the market environment are other factors that keep model accuracy at risk in the sense that it could be outright inaccurate.
A final emerging issue in this sector pertains to data security and the risk of fraud as well as cyber-attacks.
• Retail: In retail, the famous big data application is used to predict demand, create personalised marketing strategies and plan how to manage the inventory. But consumer purchasing behaviour and seasonal changes can be the significant challenges which affect the preciseness.
Furthermore, retail datasets tend to contain very personal information about the clients making the problem of data privacy a very sensitive issue as it violates clients’ rights to privacy, especially with the current Data Protection laws in place.
• Manufacturing: Predictive analytics can be useful to manufacturers particularly for maintenance prediction and for improving product quality. However, the quantity and quality of data can be both unpredictable and limited because the sensors can malfunction or, respectively, the machines themselves may be turned off.
Conversely, there are suggestions that many elements would make it difficult to provide for all influences concerning the complexities of Supply Chain management to be accorded a considerate place in the models of prediction.
However, as with any promising and innovative technology, the businesses must be able to buckle up for these pitfalls and barriers to AI-powered predictive analytics.
However, these are the challenges that can be overcome with a wider approach where bias mitigation, data security, model evaluation, and other methods of increased responsible approach to AI are used.
Implementing AI in Predictive Analytics
Applying AI as a part of the predictive analytical approaches can deliver great business value; however, it is crucial to select which tools and models are most suitable for an organisation or business in question. It is, therefore, important to start with a set of clear goals for using predictive analytics.
The decision on which Machine Learning model to choose will be predicated on common business objectives like demand forecasting, customer churn rate prediction, or risk profiling.
1. Select AI Models
• Machine Learning Models: Linear regression, decision tree, ensemble (random forest), etc. are widely used for the study of patterns in numerical data. These models are used mostly for forecasting demand and segmenting customers.
• Neural Networks: If the data to be analyzed requires more detailed patterns or it is highly sophisticated, the best system to use is the neural networks, especially the deep learning network.
CNNs and RNNs are involved in image identification tasks, and temporal sequencing and NLP; these characteristics make them fit for healthcare and finance sectors.
• Natural Language Processing (NLP): Linked NLP models transform textual data into useful information, useful in customer services and social perceptions.
2. Implement Tools and Frameworks
Many reliable tools are available for the implementation of AI predictive analysis tools including TensorFlow, PyTorch, and Scikit-Learn.
These frameworks help in the creation, training, and deployment of models, which in turn helps in creating sustainable AI software development for businesses.
3. Deploy and Monitor Models
Other important aspects remain the processes of model deployment and its live monitoring after model development. It is thus important to continue monitoring to signify that the models are up-to-date with all the incoming data to enable businesses refine parameters or retrain models.
4. Ensure Compliance and Security
Again there are concerns of data privacy and legal requirements as provided by GDPR in Europe, or HIPAA in the healthcare field when engaging AI based predictive analytics. Use secure data protection measures such as enshrinement and organizational security at the workplace.
If you want to effectively leverage these technologies, it’s essential to choose an AI development company that aligns with your business goals.
Engaging in AI software development with the right partner can significantly enhance your predictive analytics capabilities.
Choosing an Expert Partner
Сhoose a partner that has demonstrated experience in AI and predictive analytics before. This is particularly important for firms that provide AI development services that rely heavily on such techniques as machine learning, neural networks, and NLP.
For instance, Computools presents the experience in the development of artificial intelligence across various industries and provides an opportunity to solve tasks adapted to specific industry needs.
You may find out more about how our team has optimised X-ray processing via the use of AI and machine learning, or how we created an advanced platform that combines AI and data to facilitate the effective administration of patient records, medical histories, prescriptions, and scheduling.
An industry-savvy partner means that they will get acquainted with your organisation’s line of business whether it is in the health, commerce or banking sector. This convergence creates a level of familiarity that can guarantee that the AI solutions are well-aligned with the challenges facing industries, and regulations, and standards around the world.
You may learn a lot about an organisation by reviewing their case studies, including their strategies and achievements in similar projects. At its website, there should be many examples of predictive AI application in various industries including healthcare, finance, and retail, together with flexibility and effectiveness as its key approaches.
Select a product where you don’t need to look for support for one part of the process separately; your partner must cover idea generation, model development, model fine-tuning, and deployment.
Our software development company provides businesses with end-to-end support to minimise problems during integration and enhance the company’s ability to predict patterns.
Computools has rich experience in AI development and focuses on implementing unique approaches, making it an ideal choice for implementing predictive analytics.
If you require efficiencies, customer understanding, or industry trends, our team is experienced and specialised in providing customised AI solutions that can supplement individual or combined business objectives and/or industry difficulties.
We also take pride in our clients’ reviews, which reflect our commitment to delivering high-quality solutions and demonstrate our expertise in AI development.
Contact info@computools.com for all your AI development needs and business transformation inquiries.
Computools was selected through an RFP process. They were shortlisted and selected from between 5 other suppliers. Computools has worked thoroughly and timely to solve all security issues and launch as agreed. Their expertise is impressive.